The world of machine learning (ML) is a churning ocean, constantly throwing up new waves of innovation. Staying afloat in this dynamic landscape requires not just technical prowess, but also a keen awareness of the emerging trends that will shape the future of ML services. This blog dives into seven key trends that are poised to rewrite the rules of the game, from ethical considerations to quantum leaps in processing power.
Trend 1: Advancements in AI Ethics and Fairness
Gone are the days when algorithms reigned supreme without scrutiny. As ML applications infiltrate every facet of our lives, concerns about bias and fairness have taken center stage. Recent developments in ethical AI frameworks, like Microsoft’s Responsible AI Guidelines and the Montreal Declaration for Responsible AI, offer crucial guiding principles. Companies like IBM are leading the charge, embedding fairness checks into their AI development processes. This ethical wave is not just a moral imperative, but also a strategic one – studies show that bias-free AI models outperform their biased counterparts.
Trend 2: The Rise of AutoML
Remember the days when building an ML model felt like scaling Mount Everest? Enter AutoML – the automated wizard that simplifies the entire process, from data preparation to model selection. Tools like Google’s Cloud AutoML and H2O’s AutoML are making AI accessible to businesses and individuals without a team of data scientists. Roughly 61% of decision makers in companies utilizing AI said they’ve adopted autoML, and another 25% were planning to implement it that year. The future promises even greater democratization, with AutoML potentially becoming as ubiquitous as basic data analysis tools.
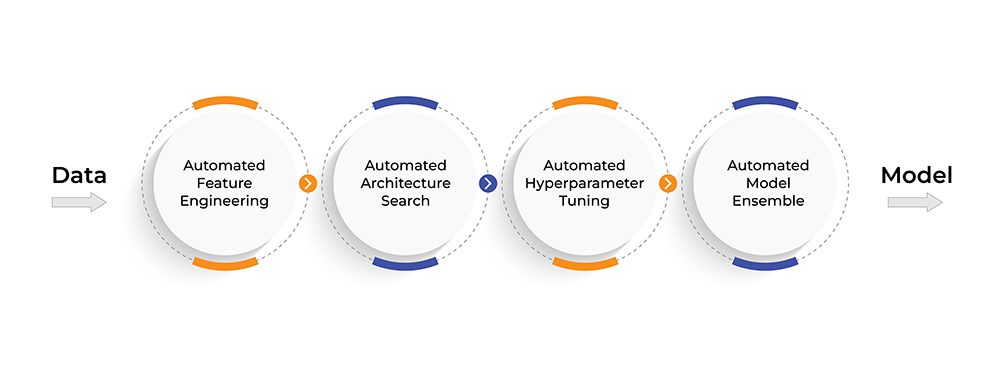
Source: Using AutoML for Time Series Forecasting – Google Research Blog
Trend 3: Machine Learning Meets Edge Computing
Imagine an AI model analyzing sensor data on a wind turbine in real-time, predicting potential malfunctions before they occur. That’s the power of edge computing – pushing ML models closer to the data source for faster, more efficient processing. Industries like manufacturing and healthcare are reaping the benefits – Siemens uses edge-based ML for predictive maintenance in factories, while hospitals are deploying similar models for real-time patient monitoring. Challenges like limited computing power and data security persist, but with advancements in edge hardware and software, the future of ML is firmly rooted at the edge.
Trend 4: AI/ML in Cybersecurity
Cybersecurity threats are evolving at breakneck speed, and traditional methods are often left in the dust. AI and ML are emerging as the new knights in shining armor, wielding powerful tools like anomaly detection and threat prediction. Companies like Deepwatch are using AI to analyze network traffic and identify malicious activity in real-time, while Darktrace’s self-learning AI detects and responds to cyberattacks autonomously. As cyber threats become more sophisticated, organizations that embrace AI-powered security will have a distinct advantage.
Trend 5: Quantum Computing’s Impact on ML
While still in its nascent stages, quantum computing holds immense potential to revolutionize machine learning. Its ability to perform complex calculations in parallel could unlock breakthroughs in areas like natural language processing and image recognition. Research projects like Google’s Sycamore quantum processor and Microsoft’s Azure Quantum Computing platform are paving the way for future applications. While widespread adoption is still years away, understanding the potential of quantum ML is crucial for staying ahead of the curve.
Trend 6: Advancements in Natural Language Processing
Natural language processing (NLP) has come a long way from rudimentary chatbots. Today, AI can understand and generate human language with remarkable nuance. Advancements like Google’s LaMDA and OpenAI’s GPT-3 are enabling machines to hold conversations, translate languages seamlessly, and even write creative content. This is transforming industries like customer service, education, and content creation. As NLP continues to evolve, the line between human and machine communication will blur even further, ushering in a new era of intelligent interaction.
Trend 7: Cross-Disciplinary Applications of ML
The power of ML isn’t limited to technology alone. When combined with other disciplines like healthcare, finance, and environmental science, it can lead to groundbreaking innovations. Imagine AI models predicting disease outbreaks with unprecedented accuracy, or analyzing financial markets to optimize investment strategies, or even monitoring environmental changes to combat climate change. These are just a glimpse of the possibilities that lie at the intersection of ML and diverse fields. Interdisciplinary collaborations will be key to unlocking the full potential of ML for the betterment of humanity.
The seven trends we’ve explored are just the tip of the iceberg. The future of ML services is brimming with possibilities, demanding continuous learning and adaptation. By understanding these emerging trends and leveraging their potential, businesses and individuals can navigate the waves of innovation and chart their course towards success in the ever-evolving landscape of machine learning.