Machine Learning’s Revolution: Crafting Personalized Learning Experiences in Education
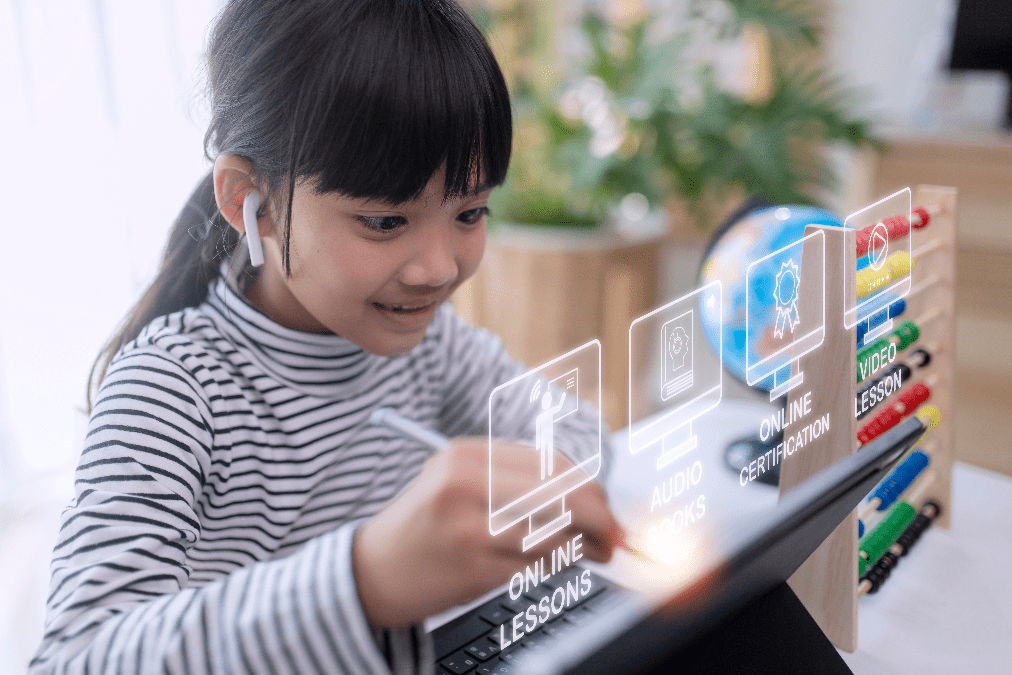
Introduction
The traditional realm of education has largely adhered to the one-size-fits-all doctrine, often sidelining the individual learning proclivities of students. However, the introduction of Machine Learning in EdTech is revolutionizing this paradigm, opening doors to bespoke educational journeys. Among all the technical advances transforming learning experiences, machine learning-powered teaching assistance is one of the top 3 most liked innovations, upvoted by 71% of higher education learners. This is because ML enables the assessment of learner behavior, interests, and personality to curate personalized learner journeys, which is the most important EdTech trend and key to boosting user engagement.
The Concept of Personalized Learning Pathways
The essence of personalized learning pathways lies in catering to the individual learning trajectories of students. It’s not merely about offering different content but tailoring the learning experience itself. Think of it as a GPS for education: instead of a singular route, multiple pathways are devised, all leading to the same destination but adjusted for the learner’s pace, preference, and proficiency. Research suggests that personalized paths make learning more targeted and efficient, which improves students’ knowledge acquisition and improves educational performance.
How ML Powers Personalization
Netflix, Sephora, and Amazon are renowned examples of how brands are leveraging personalization to boost user engagement and retention to secure revenues for the long term.
Harnessing the capabilities of Machine Learning for EdTech is akin to having a marketing tool for the EdTech firm and a supercharged teaching assistant for learners. By sifting through and analyzing vast troves of student data—ranging from their preferred learning mediums to their performance metrics—ML algorithms craft a curated educational blueprint. They not only pinpoint areas of strength but also identify knowledge gaps, allowing for targeted interventions. At the heart of Machine Learning in EdTech lies its transformative ability to accelerate the personalization process. Let’s demystify this:
- Data Collection: Every click, quiz attempt, time spent on a video, or interaction in a digital classroom is recorded, along with the chat history of students with the mentors to analyze the student’s understanding ability. This isn’t just raw data but a narraxtive of a student’s learning journey.
- Pattern Recognition: Once the data is aggregated, ML algorithms sift through these data points, identifying patterns. For example, does a student repeatedly struggle with auditory lessons but excel in visual ones? Such insights are goldmines.
- Adaptive Feedback Loops: Post pattern recognition, the system dynamically adjusts. If a student is breezing through a topic, advanced modules might be introduced. Conversely, if they’re struggling, foundational resources could be suggested.
- Predictive Analysis: Beyond real-time adaptation, ML in EdTech can foresee potential stumbling blocks. If a student’s interaction with early algebraic concepts is shaky, the system might predict challenges with more complex algebraic topics down the line, proactively offering reinforcement.
- Customized Content Delivery: It’s not just about what to teach but how to teach. Based on the learning style deduced, the content can be delivered in varied formats, be it visual, auditory, kinesthetic, or a mix, ensuring maximum absorption.
- Personalized Virtual Teaching Assistants: – AI-powered personalized tutor assistants/chatbots backed by a knowledgebase for specific subjects, which can explain topics, resolve doubts, explain and solve mathematical problems with Socratic Method of teaching according to the learning ability of the students.
Historical Context
The desire for personalized education isn’t new. Earlier methods, like differentiated instruction, were precursors to today’s advanced personalization. However, these were manual, labor-intensive, and lacked the precision of today’s technology. The emergence and subsequent integration of Machine Learning marked a watershed moment, transforming the dream of tailored education into tangible reality.
The Science Behind ML Algorithms in Education
Peel back the layers of any ML-driven educational tool, and you’ll find a blend of neural networks, deep learning, and advanced analytics at play. These algorithms don’t just track student performance; they predict it. For instance, based on a student’s interaction with a math module, the system can foresee potential challenges they might face in upcoming lessons, pre-emptively offering additional resources.
Here’s a deep dive:
- Neural Networks: Neural network-based informative content recommendations have demonstrated an accuracy of 85.2% and a recall rate of 83.7% to enrich user experiences by integrating self-attention mechanism. Applications of neural networks in EdTech are a direct translation of the underlying efficiency of deep learning to facilitate personalization. Think of these as a web of algorithms designed to recognize patterns. They interpret sensory data, filtering them through multiple layers to mimic the way a human brain processes data. In the realm of education, these networks can dissect a student’s behavior to discern their preferred learning methods.
- Deep Learning: This is a subset of ML but with greater capacities. Deep Learning algorithms use multiple layers (hence “deep”) to progressively extract higher-level features from raw input. For example, in language learning, initial layers might identify sounds, the next might recognize these sounds as words, and further layers could interpret these words’ meanings and context.
- Natural Language Processing (NLP): Especially pertinent in language-based courses, NLP helps machines understand and respond to human language. It can gauge a student’s written proficiency, offer corrective feedback, or even tailor reading materials based on the student’s vocabulary strength.
- Reinforcement Learning: This is learning by trial and error. In an educational setting, a system using reinforcement learning provides feedback (rewards or corrections) based on a student’s actions. Over time, the system learns to guide the student more effectively, nudging them towards correct solutions.
- Bias and Fairness in Algorithms: It’s critical to recognize that ML models can unintentionally perpetuate biases present in their training data. Efforts are continually made to refine these algorithms to ensure equitable educational experiences.
The Pedagogical Benefits
The advantages of ML-enhanced education are manifold. First and foremost, it breathes life into the content, making it responsive to each student. When learners feel the curriculum speaks to them directly, they’re more likely to engage deeply and consistently. This heightened engagement translates to improved comprehension and longer retention periods, ensuring a solid educational foundation. Participants agree that AIEd-led personalized education pathways stimulate mental activity and enhance their involvement while speeding up education delivery.
Potential Risks and Concerns
However, no innovation is without its set of challenges. A heavy reliance on technology could lead to a reduced emphasis on human-led instruction, which offers nuances no machine can replicate. There’s also the looming shadow of data privacy concerns. Every interaction a student has with an ML-driven tool leaves a digital footprint. Ensuring the sanctity of this data is paramount. Moreover, unchecked algorithms might inadvertently introduce biases, leading to skewed educational experiences. The key to eliminating the risks is ensuring transparency and building trust by embedding compliance within the data collection, storage, and use processes.
Future Trajectory of ML-Driven Personalization
Machine Learning, especially in EdTech, is in a constant state of evolution. As the technology becomes even more sophisticated, we’ll likely witness its permeation into niche educational segments, from special needs education to vocational training. One can envision a world where every student has a digital learning companion, fine-tuned to their needs, guiding them at every step. The next iteration of EdTech innovation will be “evidence-powered.” It will focus on differentiated instruction that will be self-improving, empathetic, immersive, and backed by data-driven insights, courtesy machine learning and analytics. This is bound to facilitate personalization at scale.
As we stand at the crossroads of traditional education and tech-driven instruction, it’s evident that Machine Learning isn’t just an add-on; it’s integral to the future educational fabric. As we forge ahead, the harmonious melding of human touch with technological precision will shape the educational experiences of tomorrow.
News & Insights
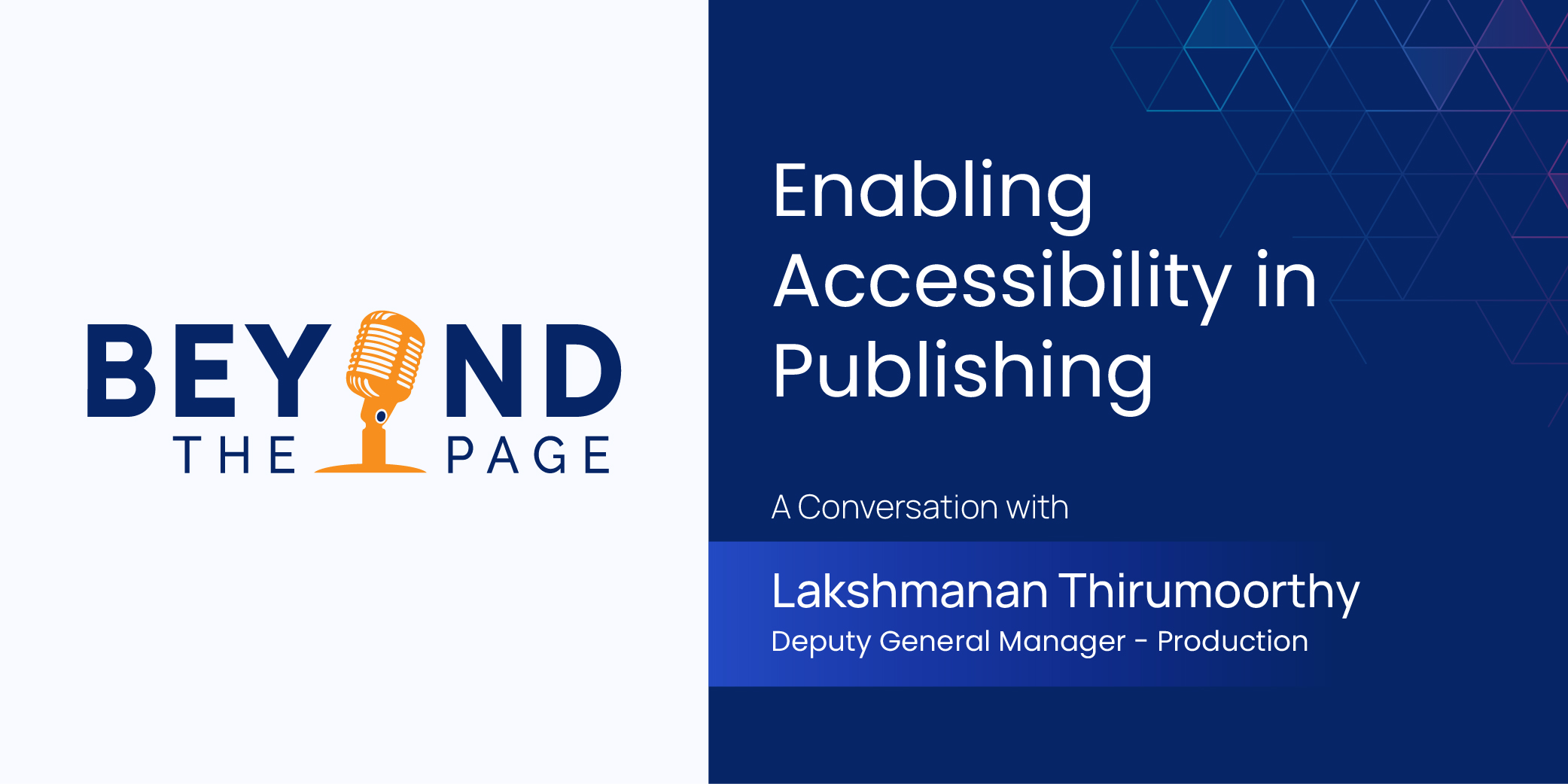
Enabling Accessibility in Scholarly Publishing – A Conversation with Lakshmanan Thirumoorthy
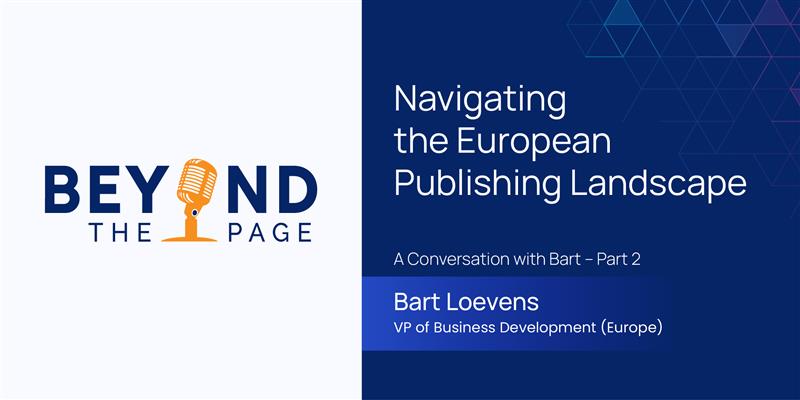
Navigating the European Publishing Landscape – A Conversation with Bart – Part 2
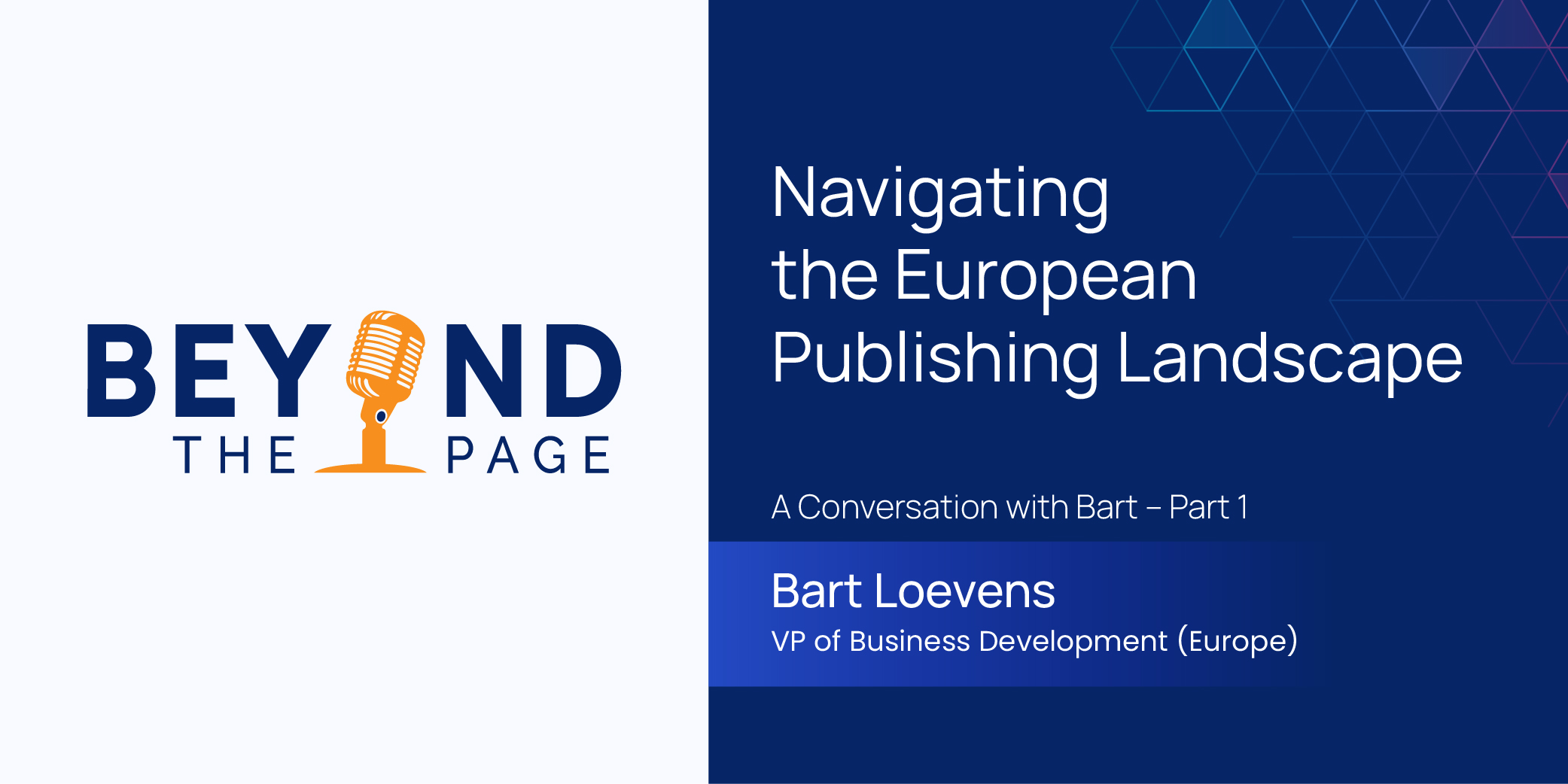